Key-text spotting in documentary videos using Adaboost
Identifieur interne :
000446 ( PascalFrancis/Curation );
précédent :
000445;
suivant :
000447
Key-text spotting in documentary videos using Adaboost
Auteurs : M. Lalonde [
Canada] ;
L. Gagnon [
Canada]
Source :
-
Proceedings of SPIE, the International Society for Optical Engineering [ 0277-786X ] ; 2006.
RBID : Pascal:07-0365691
Descripteurs français
English descriptors
Abstract
This paper presents a method for spotting key-text in videos, based on a cascade of classifiers trained with Adaboost. The video is first reduced to a set of key-frames. Each key-frame is then analyzed for its text content. Text spotting is performed by scanning the image with a variable-size window (to account for scale) within which simple features (mean/variance of grayscale values and x/y derivatives) are extracted in various sub-areas. Training builds classifiers using the most discriminant spatial combinations of features for text detection. The text-spotting module outputs a decision map of the size of the input key-frame showing regions of interest that may contain text suitable for recognition by an OCR system. Performance is measured against a dataset of 147 key-frames extracted from 22 documentary films of the National Film Board (NFB) of Canada. A detection rate of 97% is obtained with relatively few false alarms.
pA |
A01 | 01 | 1 | | @0 0277-786X |
---|
A05 | | | | @2 6064 |
---|
A08 | 01 | 1 | ENG | @1 Key-text spotting in documentary videos using Adaboost |
---|
A09 | 01 | 1 | ENG | @1 Image processing : algorithms and systems, neural networks, and machine learning : 16-18 January 2006, San Jose, California, USA |
---|
A11 | 01 | 1 | | @1 LALONDE (M.) |
---|
A11 | 02 | 1 | | @1 GAGNON (L.) |
---|
A12 | 01 | 1 | | @1 DOUGHERTY (Edward R.) @9 ed. |
---|
A14 | 01 | | | @1 R&D Department, Computer Research Institute of Montreal (CRIM), 550 Sherbrooke West, Suite 100 @2 Montreal, QC, H3A 1B9 @3 CAN @Z 1 aut. @Z 2 aut. |
---|
A18 | 01 | 1 | | @1 IS&T--The Society for Imaging Science and Technology @3 USA @9 org-cong. |
---|
A18 | 02 | 1 | | @1 Society of photo-optical instrumentation engineers @3 USA @9 org-cong. |
---|
A20 | | | | @2 60641N.1-60641N.8 |
---|
A21 | | | | @1 2006 |
---|
A23 | 01 | | | @0 ENG |
---|
A26 | 01 | | | @0 0-8194-6104-0 |
---|
A43 | 01 | | | @1 INIST @2 21760 @5 354000153558040510 |
---|
A44 | | | | @0 0000 @1 © 2007 INIST-CNRS. All rights reserved. |
---|
A45 | | | | @0 17 ref. |
---|
A47 | 01 | 1 | | @0 07-0365691 |
---|
A60 | | | | @1 P @2 C |
---|
A61 | | | | @0 A |
---|
A64 | 01 | 1 | | @0 Proceedings of SPIE, the International Society for Optical Engineering |
---|
A66 | 01 | | | @0 USA |
---|
C01 | 01 | | ENG | @0 This paper presents a method for spotting key-text in videos, based on a cascade of classifiers trained with Adaboost. The video is first reduced to a set of key-frames. Each key-frame is then analyzed for its text content. Text spotting is performed by scanning the image with a variable-size window (to account for scale) within which simple features (mean/variance of grayscale values and x/y derivatives) are extracted in various sub-areas. Training builds classifiers using the most discriminant spatial combinations of features for text detection. The text-spotting module outputs a decision map of the size of the input key-frame showing regions of interest that may contain text suitable for recognition by an OCR system. Performance is measured against a dataset of 147 key-frames extracted from 22 documentary films of the National Film Board (NFB) of Canada. A detection rate of 97% is obtained with relatively few false alarms. |
---|
C02 | 01 | 3 | | @0 001B00G05P |
---|
C02 | 02 | 3 | | @0 001B40B30V |
---|
C03 | 01 | 3 | FRE | @0 Traitement image @5 61 |
---|
C03 | 01 | 3 | ENG | @0 Image processing @5 61 |
---|
C03 | 02 | 3 | FRE | @0 0705P @4 INC @5 83 |
---|
C03 | 03 | 3 | FRE | @0 4230V @4 INC @5 91 |
---|
N21 | | | | @1 239 |
---|
N44 | 01 | | | @1 OTO |
---|
N82 | | | | @1 OTO |
---|
|
pR |
A30 | 01 | 1 | ENG | @1 Image processing @3 USA @4 2006 |
---|
|
Links toward previous steps (curation, corpus...)
- to stream PascalFrancis, to step Corpus: Pour aller vers cette notice dans l'étape Curation :000340
Links to Exploration step
Pascal:07-0365691
Le document en format XML
<record><TEI><teiHeader><fileDesc><titleStmt><title xml:lang="en" level="a">Key-text spotting in documentary videos using Adaboost</title>
<author><name sortKey="Lalonde, M" sort="Lalonde, M" uniqKey="Lalonde M" first="M." last="Lalonde">M. Lalonde</name>
<affiliation wicri:level="1"><inist:fA14 i1="01"><s1>R&D Department, Computer Research Institute of Montreal (CRIM), 550 Sherbrooke West, Suite 100</s1>
<s2>Montreal, QC, H3A 1B9</s2>
<s3>CAN</s3>
<sZ>1 aut.</sZ>
<sZ>2 aut.</sZ>
</inist:fA14>
<country>Canada</country>
</affiliation>
</author>
<author><name sortKey="Gagnon, L" sort="Gagnon, L" uniqKey="Gagnon L" first="L." last="Gagnon">L. Gagnon</name>
<affiliation wicri:level="1"><inist:fA14 i1="01"><s1>R&D Department, Computer Research Institute of Montreal (CRIM), 550 Sherbrooke West, Suite 100</s1>
<s2>Montreal, QC, H3A 1B9</s2>
<s3>CAN</s3>
<sZ>1 aut.</sZ>
<sZ>2 aut.</sZ>
</inist:fA14>
<country>Canada</country>
</affiliation>
</author>
</titleStmt>
<publicationStmt><idno type="wicri:source">INIST</idno>
<idno type="inist">07-0365691</idno>
<date when="2006">2006</date>
<idno type="stanalyst">PASCAL 07-0365691 INIST</idno>
<idno type="RBID">Pascal:07-0365691</idno>
<idno type="wicri:Area/PascalFrancis/Corpus">000340</idno>
<idno type="wicri:Area/PascalFrancis/Curation">000446</idno>
</publicationStmt>
<sourceDesc><biblStruct><analytic><title xml:lang="en" level="a">Key-text spotting in documentary videos using Adaboost</title>
<author><name sortKey="Lalonde, M" sort="Lalonde, M" uniqKey="Lalonde M" first="M." last="Lalonde">M. Lalonde</name>
<affiliation wicri:level="1"><inist:fA14 i1="01"><s1>R&D Department, Computer Research Institute of Montreal (CRIM), 550 Sherbrooke West, Suite 100</s1>
<s2>Montreal, QC, H3A 1B9</s2>
<s3>CAN</s3>
<sZ>1 aut.</sZ>
<sZ>2 aut.</sZ>
</inist:fA14>
<country>Canada</country>
</affiliation>
</author>
<author><name sortKey="Gagnon, L" sort="Gagnon, L" uniqKey="Gagnon L" first="L." last="Gagnon">L. Gagnon</name>
<affiliation wicri:level="1"><inist:fA14 i1="01"><s1>R&D Department, Computer Research Institute of Montreal (CRIM), 550 Sherbrooke West, Suite 100</s1>
<s2>Montreal, QC, H3A 1B9</s2>
<s3>CAN</s3>
<sZ>1 aut.</sZ>
<sZ>2 aut.</sZ>
</inist:fA14>
<country>Canada</country>
</affiliation>
</author>
</analytic>
<series><title level="j" type="main">Proceedings of SPIE, the International Society for Optical Engineering</title>
<idno type="ISSN">0277-786X</idno>
<imprint><date when="2006">2006</date>
</imprint>
</series>
</biblStruct>
</sourceDesc>
<seriesStmt><title level="j" type="main">Proceedings of SPIE, the International Society for Optical Engineering</title>
<idno type="ISSN">0277-786X</idno>
</seriesStmt>
</fileDesc>
<profileDesc><textClass><keywords scheme="KwdEn" xml:lang="en"><term>Image processing</term>
</keywords>
<keywords scheme="Pascal" xml:lang="fr"><term>Traitement image</term>
<term>0705P</term>
<term>4230V</term>
</keywords>
</textClass>
</profileDesc>
</teiHeader>
<front><div type="abstract" xml:lang="en">This paper presents a method for spotting key-text in videos, based on a cascade of classifiers trained with Adaboost. The video is first reduced to a set of key-frames. Each key-frame is then analyzed for its text content. Text spotting is performed by scanning the image with a variable-size window (to account for scale) within which simple features (mean/variance of grayscale values and x/y derivatives) are extracted in various sub-areas. Training builds classifiers using the most discriminant spatial combinations of features for text detection. The text-spotting module outputs a decision map of the size of the input key-frame showing regions of interest that may contain text suitable for recognition by an OCR system. Performance is measured against a dataset of 147 key-frames extracted from 22 documentary films of the National Film Board (NFB) of Canada. A detection rate of 97% is obtained with relatively few false alarms.</div>
</front>
</TEI>
<inist><standard h6="B"><pA><fA01 i1="01" i2="1"><s0>0277-786X</s0>
</fA01>
<fA05><s2>6064</s2>
</fA05>
<fA08 i1="01" i2="1" l="ENG"><s1>Key-text spotting in documentary videos using Adaboost</s1>
</fA08>
<fA09 i1="01" i2="1" l="ENG"><s1>Image processing : algorithms and systems, neural networks, and machine learning : 16-18 January 2006, San Jose, California, USA</s1>
</fA09>
<fA11 i1="01" i2="1"><s1>LALONDE (M.)</s1>
</fA11>
<fA11 i1="02" i2="1"><s1>GAGNON (L.)</s1>
</fA11>
<fA12 i1="01" i2="1"><s1>DOUGHERTY (Edward R.)</s1>
<s9>ed.</s9>
</fA12>
<fA14 i1="01"><s1>R&D Department, Computer Research Institute of Montreal (CRIM), 550 Sherbrooke West, Suite 100</s1>
<s2>Montreal, QC, H3A 1B9</s2>
<s3>CAN</s3>
<sZ>1 aut.</sZ>
<sZ>2 aut.</sZ>
</fA14>
<fA18 i1="01" i2="1"><s1>IS&T--The Society for Imaging Science and Technology</s1>
<s3>USA</s3>
<s9>org-cong.</s9>
</fA18>
<fA18 i1="02" i2="1"><s1>Society of photo-optical instrumentation engineers</s1>
<s3>USA</s3>
<s9>org-cong.</s9>
</fA18>
<fA20><s2>60641N.1-60641N.8</s2>
</fA20>
<fA21><s1>2006</s1>
</fA21>
<fA23 i1="01"><s0>ENG</s0>
</fA23>
<fA26 i1="01"><s0>0-8194-6104-0</s0>
</fA26>
<fA43 i1="01"><s1>INIST</s1>
<s2>21760</s2>
<s5>354000153558040510</s5>
</fA43>
<fA44><s0>0000</s0>
<s1>© 2007 INIST-CNRS. All rights reserved.</s1>
</fA44>
<fA45><s0>17 ref.</s0>
</fA45>
<fA47 i1="01" i2="1"><s0>07-0365691</s0>
</fA47>
<fA60><s1>P</s1>
<s2>C</s2>
</fA60>
<fA64 i1="01" i2="1"><s0>Proceedings of SPIE, the International Society for Optical Engineering</s0>
</fA64>
<fA66 i1="01"><s0>USA</s0>
</fA66>
<fC01 i1="01" l="ENG"><s0>This paper presents a method for spotting key-text in videos, based on a cascade of classifiers trained with Adaboost. The video is first reduced to a set of key-frames. Each key-frame is then analyzed for its text content. Text spotting is performed by scanning the image with a variable-size window (to account for scale) within which simple features (mean/variance of grayscale values and x/y derivatives) are extracted in various sub-areas. Training builds classifiers using the most discriminant spatial combinations of features for text detection. The text-spotting module outputs a decision map of the size of the input key-frame showing regions of interest that may contain text suitable for recognition by an OCR system. Performance is measured against a dataset of 147 key-frames extracted from 22 documentary films of the National Film Board (NFB) of Canada. A detection rate of 97% is obtained with relatively few false alarms.</s0>
</fC01>
<fC02 i1="01" i2="3"><s0>001B00G05P</s0>
</fC02>
<fC02 i1="02" i2="3"><s0>001B40B30V</s0>
</fC02>
<fC03 i1="01" i2="3" l="FRE"><s0>Traitement image</s0>
<s5>61</s5>
</fC03>
<fC03 i1="01" i2="3" l="ENG"><s0>Image processing</s0>
<s5>61</s5>
</fC03>
<fC03 i1="02" i2="3" l="FRE"><s0>0705P</s0>
<s4>INC</s4>
<s5>83</s5>
</fC03>
<fC03 i1="03" i2="3" l="FRE"><s0>4230V</s0>
<s4>INC</s4>
<s5>91</s5>
</fC03>
<fN21><s1>239</s1>
</fN21>
<fN44 i1="01"><s1>OTO</s1>
</fN44>
<fN82><s1>OTO</s1>
</fN82>
</pA>
<pR><fA30 i1="01" i2="1" l="ENG"><s1>Image processing</s1>
<s3>USA</s3>
<s4>2006</s4>
</fA30>
</pR>
</standard>
</inist>
</record>
Pour manipuler ce document sous Unix (Dilib)
EXPLOR_STEP=$WICRI_ROOT/Ticri/CIDE/explor/OcrV1/Data/PascalFrancis/Curation
HfdSelect -h $EXPLOR_STEP/biblio.hfd -nk 000446 | SxmlIndent | more
Ou
HfdSelect -h $EXPLOR_AREA/Data/PascalFrancis/Curation/biblio.hfd -nk 000446 | SxmlIndent | more
Pour mettre un lien sur cette page dans le réseau Wicri
{{Explor lien
|wiki= Ticri/CIDE
|area= OcrV1
|flux= PascalFrancis
|étape= Curation
|type= RBID
|clé= Pascal:07-0365691
|texte= Key-text spotting in documentary videos using Adaboost
}}
| This area was generated with Dilib version V0.6.32. Data generation: Sat Nov 11 16:53:45 2017. Site generation: Mon Mar 11 23:15:16 2024 | 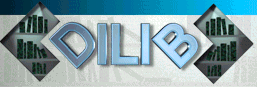 |