Hybrid OCR combination for ancient documents
Identifieur interne :
000449 ( PascalFrancis/Corpus );
précédent :
000448;
suivant :
000450
Hybrid OCR combination for ancient documents
Auteurs : Hubert Cecotti ;
Abdel BelaidSource :
-
Lecture notes in computer science [ 0302-9743 ] ; 2005.
RBID : Pascal:05-0391622
Descripteurs français
English descriptors
Abstract
Commercial Optical Character Recognition (OCR) have at lot improved in the last few years. Their outstanding ability to process different kinds of documents is their main quality. However, their generality can also be an issue, as they cannot recognize perfectly documents far from the average present-day documents. We propose in this paper a system combining several OCRs and a specialized ICR (Intelligent Character Recognition) based on a convolutional neural network to complement them. Instead of just performing several OCRs in parallel and applying a fusing rule on the results, a specialized neural network with an adaptive topology is added to complement the OCRs, in function of the OCRs errors. This system has been tested on ancient documents containing old characters and old fonts not used in contemporary documents. The OCRs combination increases the recognition of about 3% whereas the ICR improves the recognition of rejected characters of more than 5%.
Notice en format standard (ISO 2709)
Pour connaître la documentation sur le format Inist Standard.
pA |
A01 | 01 | 1 | | @0 0302-9743 |
---|
A05 | | | | @2 3686 |
---|
A08 | 01 | 1 | ENG | @1 Hybrid OCR combination for ancient documents |
---|
A09 | 01 | 1 | ENG | @1 Pattern recognition and data mining : Bath, 22-25 august 2005 |
---|
A11 | 01 | 1 | | @1 CECOTTI (Hubert) |
---|
A11 | 02 | 1 | | @1 BELAID (Abdel) |
---|
A12 | 01 | 1 | | @1 SINGH (Sameer) @9 ed. |
---|
A12 | 02 | 1 | | @1 SINGH (Maneesha) @9 ed. |
---|
A12 | 03 | 1 | | @1 APTE (Chid) @9 ed. |
---|
A12 | 04 | 1 | | @1 PERNER (Petra) @9 ed. |
---|
A14 | 01 | | | @1 READ Group, LORIA/CNRS, Campus Scientifique BP 239 @2 54506 Vandoeuvre-les-Nancy @3 FRA @Z 1 aut. @Z 2 aut. |
---|
A20 | | | | @2 Part I, 646-653 |
---|
A21 | | | | @1 2005 |
---|
A23 | 01 | | | @0 ENG |
---|
A26 | 01 | | | @0 3-540-28757-4 |
---|
A43 | 01 | | | @1 INIST @2 16343 @5 354000124412760710 |
---|
A44 | | | | @0 0000 @1 © 2005 INIST-CNRS. All rights reserved. |
---|
A45 | | | | @0 16 ref. |
---|
A47 | 01 | 1 | | @0 05-0391622 |
---|
A60 | | | | @1 P @2 C |
---|
A61 | | | | @0 A |
---|
A64 | 01 | 1 | | @0 Lecture notes in computer science |
---|
A66 | 01 | | | @0 DEU |
---|
C01 | 01 | | ENG | @0 Commercial Optical Character Recognition (OCR) have at lot improved in the last few years. Their outstanding ability to process different kinds of documents is their main quality. However, their generality can also be an issue, as they cannot recognize perfectly documents far from the average present-day documents. We propose in this paper a system combining several OCRs and a specialized ICR (Intelligent Character Recognition) based on a convolutional neural network to complement them. Instead of just performing several OCRs in parallel and applying a fusing rule on the results, a specialized neural network with an adaptive topology is added to complement the OCRs, in function of the OCRs errors. This system has been tested on ancient documents containing old characters and old fonts not used in contemporary documents. The OCRs combination increases the recognition of about 3% whereas the ICR improves the recognition of rejected characters of more than 5%. |
---|
C02 | 01 | X | | @0 001D02B07B |
---|
C03 | 01 | X | FRE | @0 Fouille donnée @5 01 |
---|
C03 | 01 | X | ENG | @0 Data mining @5 01 |
---|
C03 | 01 | X | SPA | @0 Busca dato @5 01 |
---|
C03 | 02 | X | FRE | @0 Reconnaissance caractère @5 06 |
---|
C03 | 02 | X | ENG | @0 Character recognition @5 06 |
---|
C03 | 02 | X | SPA | @0 Reconocimiento carácter @5 06 |
---|
C03 | 03 | X | FRE | @0 Reconnaissance optique caractère @5 07 |
---|
C03 | 03 | X | ENG | @0 Optical character recognition @5 07 |
---|
C03 | 03 | X | SPA | @0 Reconocimento óptico de caracteres @5 07 |
---|
C03 | 04 | X | FRE | @0 Reconnaissance forme @5 08 |
---|
C03 | 04 | X | ENG | @0 Pattern recognition @5 08 |
---|
C03 | 04 | X | SPA | @0 Reconocimiento patrón @5 08 |
---|
C03 | 05 | 3 | FRE | @0 Topologie circuit @5 18 |
---|
C03 | 05 | 3 | ENG | @0 Network topology @5 18 |
---|
C03 | 06 | X | FRE | @0 Réseau neuronal @5 23 |
---|
C03 | 06 | X | ENG | @0 Neural network @5 23 |
---|
C03 | 06 | X | SPA | @0 Red neuronal @5 23 |
---|
C03 | 07 | X | FRE | @0 Méthode adaptative @5 24 |
---|
C03 | 07 | X | ENG | @0 Adaptive method @5 24 |
---|
C03 | 07 | X | SPA | @0 Método adaptativo @5 24 |
---|
C03 | 08 | X | FRE | @0 Fonction erreur @5 25 |
---|
C03 | 08 | X | ENG | @0 Error function @5 25 |
---|
C03 | 08 | X | SPA | @0 Función error @5 25 |
---|
N21 | | | | @1 276 |
---|
N44 | 01 | | | @1 OTO |
---|
N82 | | | | @1 OTO |
---|
|
pR |
A30 | 01 | 1 | ENG | @1 ICAPR : international conference on advances in pattern recognition @3 Bath GBR @4 2005-08-22 |
---|
|
Format Inist (serveur)
NO : | PASCAL 05-0391622 INIST |
ET : | Hybrid OCR combination for ancient documents |
AU : | CECOTTI (Hubert); BELAID (Abdel); SINGH (Sameer); SINGH (Maneesha); APTE (Chid); PERNER (Petra) |
AF : | READ Group, LORIA/CNRS, Campus Scientifique BP 239/54506 Vandoeuvre-les-Nancy/France (1 aut., 2 aut.) |
DT : | Publication en série; Congrès; Niveau analytique |
SO : | Lecture notes in computer science; ISSN 0302-9743; Allemagne; Da. 2005; Vol. 3686; Part I, 646-653; Bibl. 16 ref. |
LA : | Anglais |
EA : | Commercial Optical Character Recognition (OCR) have at lot improved in the last few years. Their outstanding ability to process different kinds of documents is their main quality. However, their generality can also be an issue, as they cannot recognize perfectly documents far from the average present-day documents. We propose in this paper a system combining several OCRs and a specialized ICR (Intelligent Character Recognition) based on a convolutional neural network to complement them. Instead of just performing several OCRs in parallel and applying a fusing rule on the results, a specialized neural network with an adaptive topology is added to complement the OCRs, in function of the OCRs errors. This system has been tested on ancient documents containing old characters and old fonts not used in contemporary documents. The OCRs combination increases the recognition of about 3% whereas the ICR improves the recognition of rejected characters of more than 5%. |
CC : | 001D02B07B |
FD : | Fouille donnée; Reconnaissance caractère; Reconnaissance optique caractère; Reconnaissance forme; Topologie circuit; Réseau neuronal; Méthode adaptative; Fonction erreur |
ED : | Data mining; Character recognition; Optical character recognition; Pattern recognition; Network topology; Neural network; Adaptive method; Error function |
SD : | Busca dato; Reconocimiento carácter; Reconocimento óptico de caracteres; Reconocimiento patrón; Red neuronal; Método adaptativo; Función error |
LO : | INIST-16343.354000124412760710 |
ID : | 05-0391622 |
Links to Exploration step
Pascal:05-0391622
Le document en format XML
<record><TEI><teiHeader><fileDesc><titleStmt><title xml:lang="en" level="a">Hybrid OCR combination for ancient documents</title>
<author><name sortKey="Cecotti, Hubert" sort="Cecotti, Hubert" uniqKey="Cecotti H" first="Hubert" last="Cecotti">Hubert Cecotti</name>
<affiliation><inist:fA14 i1="01"><s1>READ Group, LORIA/CNRS, Campus Scientifique BP 239</s1>
<s2>54506 Vandoeuvre-les-Nancy</s2>
<s3>FRA</s3>
<sZ>1 aut.</sZ>
<sZ>2 aut.</sZ>
</inist:fA14>
</affiliation>
</author>
<author><name sortKey="Belaid, Abdel" sort="Belaid, Abdel" uniqKey="Belaid A" first="Abdel" last="Belaid">Abdel Belaid</name>
<affiliation><inist:fA14 i1="01"><s1>READ Group, LORIA/CNRS, Campus Scientifique BP 239</s1>
<s2>54506 Vandoeuvre-les-Nancy</s2>
<s3>FRA</s3>
<sZ>1 aut.</sZ>
<sZ>2 aut.</sZ>
</inist:fA14>
</affiliation>
</author>
</titleStmt>
<publicationStmt><idno type="wicri:source">INIST</idno>
<idno type="inist">05-0391622</idno>
<date when="2005">2005</date>
<idno type="stanalyst">PASCAL 05-0391622 INIST</idno>
<idno type="RBID">Pascal:05-0391622</idno>
<idno type="wicri:Area/PascalFrancis/Corpus">000449</idno>
</publicationStmt>
<sourceDesc><biblStruct><analytic><title xml:lang="en" level="a">Hybrid OCR combination for ancient documents</title>
<author><name sortKey="Cecotti, Hubert" sort="Cecotti, Hubert" uniqKey="Cecotti H" first="Hubert" last="Cecotti">Hubert Cecotti</name>
<affiliation><inist:fA14 i1="01"><s1>READ Group, LORIA/CNRS, Campus Scientifique BP 239</s1>
<s2>54506 Vandoeuvre-les-Nancy</s2>
<s3>FRA</s3>
<sZ>1 aut.</sZ>
<sZ>2 aut.</sZ>
</inist:fA14>
</affiliation>
</author>
<author><name sortKey="Belaid, Abdel" sort="Belaid, Abdel" uniqKey="Belaid A" first="Abdel" last="Belaid">Abdel Belaid</name>
<affiliation><inist:fA14 i1="01"><s1>READ Group, LORIA/CNRS, Campus Scientifique BP 239</s1>
<s2>54506 Vandoeuvre-les-Nancy</s2>
<s3>FRA</s3>
<sZ>1 aut.</sZ>
<sZ>2 aut.</sZ>
</inist:fA14>
</affiliation>
</author>
</analytic>
<series><title level="j" type="main">Lecture notes in computer science</title>
<idno type="ISSN">0302-9743</idno>
<imprint><date when="2005">2005</date>
</imprint>
</series>
</biblStruct>
</sourceDesc>
<seriesStmt><title level="j" type="main">Lecture notes in computer science</title>
<idno type="ISSN">0302-9743</idno>
</seriesStmt>
</fileDesc>
<profileDesc><textClass><keywords scheme="KwdEn" xml:lang="en"><term>Adaptive method</term>
<term>Character recognition</term>
<term>Data mining</term>
<term>Error function</term>
<term>Network topology</term>
<term>Neural network</term>
<term>Optical character recognition</term>
<term>Pattern recognition</term>
</keywords>
<keywords scheme="Pascal" xml:lang="fr"><term>Fouille donnée</term>
<term>Reconnaissance caractère</term>
<term>Reconnaissance optique caractère</term>
<term>Reconnaissance forme</term>
<term>Topologie circuit</term>
<term>Réseau neuronal</term>
<term>Méthode adaptative</term>
<term>Fonction erreur</term>
</keywords>
</textClass>
</profileDesc>
</teiHeader>
<front><div type="abstract" xml:lang="en">Commercial Optical Character Recognition (OCR) have at lot improved in the last few years. Their outstanding ability to process different kinds of documents is their main quality. However, their generality can also be an issue, as they cannot recognize perfectly documents far from the average present-day documents. We propose in this paper a system combining several OCRs and a specialized ICR (Intelligent Character Recognition) based on a convolutional neural network to complement them. Instead of just performing several OCRs in parallel and applying a fusing rule on the results, a specialized neural network with an adaptive topology is added to complement the OCRs, in function of the OCRs errors. This system has been tested on ancient documents containing old characters and old fonts not used in contemporary documents. The OCRs combination increases the recognition of about 3% whereas the ICR improves the recognition of rejected characters of more than 5%.</div>
</front>
</TEI>
<inist><standard h6="B"><pA><fA01 i1="01" i2="1"><s0>0302-9743</s0>
</fA01>
<fA05><s2>3686</s2>
</fA05>
<fA08 i1="01" i2="1" l="ENG"><s1>Hybrid OCR combination for ancient documents</s1>
</fA08>
<fA09 i1="01" i2="1" l="ENG"><s1>Pattern recognition and data mining : Bath, 22-25 august 2005</s1>
</fA09>
<fA11 i1="01" i2="1"><s1>CECOTTI (Hubert)</s1>
</fA11>
<fA11 i1="02" i2="1"><s1>BELAID (Abdel)</s1>
</fA11>
<fA12 i1="01" i2="1"><s1>SINGH (Sameer)</s1>
<s9>ed.</s9>
</fA12>
<fA12 i1="02" i2="1"><s1>SINGH (Maneesha)</s1>
<s9>ed.</s9>
</fA12>
<fA12 i1="03" i2="1"><s1>APTE (Chid)</s1>
<s9>ed.</s9>
</fA12>
<fA12 i1="04" i2="1"><s1>PERNER (Petra)</s1>
<s9>ed.</s9>
</fA12>
<fA14 i1="01"><s1>READ Group, LORIA/CNRS, Campus Scientifique BP 239</s1>
<s2>54506 Vandoeuvre-les-Nancy</s2>
<s3>FRA</s3>
<sZ>1 aut.</sZ>
<sZ>2 aut.</sZ>
</fA14>
<fA20><s2>Part I, 646-653</s2>
</fA20>
<fA21><s1>2005</s1>
</fA21>
<fA23 i1="01"><s0>ENG</s0>
</fA23>
<fA26 i1="01"><s0>3-540-28757-4</s0>
</fA26>
<fA43 i1="01"><s1>INIST</s1>
<s2>16343</s2>
<s5>354000124412760710</s5>
</fA43>
<fA44><s0>0000</s0>
<s1>© 2005 INIST-CNRS. All rights reserved.</s1>
</fA44>
<fA45><s0>16 ref.</s0>
</fA45>
<fA47 i1="01" i2="1"><s0>05-0391622</s0>
</fA47>
<fA60><s1>P</s1>
<s2>C</s2>
</fA60>
<fA64 i1="01" i2="1"><s0>Lecture notes in computer science</s0>
</fA64>
<fA66 i1="01"><s0>DEU</s0>
</fA66>
<fC01 i1="01" l="ENG"><s0>Commercial Optical Character Recognition (OCR) have at lot improved in the last few years. Their outstanding ability to process different kinds of documents is their main quality. However, their generality can also be an issue, as they cannot recognize perfectly documents far from the average present-day documents. We propose in this paper a system combining several OCRs and a specialized ICR (Intelligent Character Recognition) based on a convolutional neural network to complement them. Instead of just performing several OCRs in parallel and applying a fusing rule on the results, a specialized neural network with an adaptive topology is added to complement the OCRs, in function of the OCRs errors. This system has been tested on ancient documents containing old characters and old fonts not used in contemporary documents. The OCRs combination increases the recognition of about 3% whereas the ICR improves the recognition of rejected characters of more than 5%.</s0>
</fC01>
<fC02 i1="01" i2="X"><s0>001D02B07B</s0>
</fC02>
<fC03 i1="01" i2="X" l="FRE"><s0>Fouille donnée</s0>
<s5>01</s5>
</fC03>
<fC03 i1="01" i2="X" l="ENG"><s0>Data mining</s0>
<s5>01</s5>
</fC03>
<fC03 i1="01" i2="X" l="SPA"><s0>Busca dato</s0>
<s5>01</s5>
</fC03>
<fC03 i1="02" i2="X" l="FRE"><s0>Reconnaissance caractère</s0>
<s5>06</s5>
</fC03>
<fC03 i1="02" i2="X" l="ENG"><s0>Character recognition</s0>
<s5>06</s5>
</fC03>
<fC03 i1="02" i2="X" l="SPA"><s0>Reconocimiento carácter</s0>
<s5>06</s5>
</fC03>
<fC03 i1="03" i2="X" l="FRE"><s0>Reconnaissance optique caractère</s0>
<s5>07</s5>
</fC03>
<fC03 i1="03" i2="X" l="ENG"><s0>Optical character recognition</s0>
<s5>07</s5>
</fC03>
<fC03 i1="03" i2="X" l="SPA"><s0>Reconocimento óptico de caracteres</s0>
<s5>07</s5>
</fC03>
<fC03 i1="04" i2="X" l="FRE"><s0>Reconnaissance forme</s0>
<s5>08</s5>
</fC03>
<fC03 i1="04" i2="X" l="ENG"><s0>Pattern recognition</s0>
<s5>08</s5>
</fC03>
<fC03 i1="04" i2="X" l="SPA"><s0>Reconocimiento patrón</s0>
<s5>08</s5>
</fC03>
<fC03 i1="05" i2="3" l="FRE"><s0>Topologie circuit</s0>
<s5>18</s5>
</fC03>
<fC03 i1="05" i2="3" l="ENG"><s0>Network topology</s0>
<s5>18</s5>
</fC03>
<fC03 i1="06" i2="X" l="FRE"><s0>Réseau neuronal</s0>
<s5>23</s5>
</fC03>
<fC03 i1="06" i2="X" l="ENG"><s0>Neural network</s0>
<s5>23</s5>
</fC03>
<fC03 i1="06" i2="X" l="SPA"><s0>Red neuronal</s0>
<s5>23</s5>
</fC03>
<fC03 i1="07" i2="X" l="FRE"><s0>Méthode adaptative</s0>
<s5>24</s5>
</fC03>
<fC03 i1="07" i2="X" l="ENG"><s0>Adaptive method</s0>
<s5>24</s5>
</fC03>
<fC03 i1="07" i2="X" l="SPA"><s0>Método adaptativo</s0>
<s5>24</s5>
</fC03>
<fC03 i1="08" i2="X" l="FRE"><s0>Fonction erreur</s0>
<s5>25</s5>
</fC03>
<fC03 i1="08" i2="X" l="ENG"><s0>Error function</s0>
<s5>25</s5>
</fC03>
<fC03 i1="08" i2="X" l="SPA"><s0>Función error</s0>
<s5>25</s5>
</fC03>
<fN21><s1>276</s1>
</fN21>
<fN44 i1="01"><s1>OTO</s1>
</fN44>
<fN82><s1>OTO</s1>
</fN82>
</pA>
<pR><fA30 i1="01" i2="1" l="ENG"><s1>ICAPR : international conference on advances in pattern recognition</s1>
<s3>Bath GBR</s3>
<s4>2005-08-22</s4>
</fA30>
</pR>
</standard>
<server><NO>PASCAL 05-0391622 INIST</NO>
<ET>Hybrid OCR combination for ancient documents</ET>
<AU>CECOTTI (Hubert); BELAID (Abdel); SINGH (Sameer); SINGH (Maneesha); APTE (Chid); PERNER (Petra)</AU>
<AF>READ Group, LORIA/CNRS, Campus Scientifique BP 239/54506 Vandoeuvre-les-Nancy/France (1 aut., 2 aut.)</AF>
<DT>Publication en série; Congrès; Niveau analytique</DT>
<SO>Lecture notes in computer science; ISSN 0302-9743; Allemagne; Da. 2005; Vol. 3686; Part I, 646-653; Bibl. 16 ref.</SO>
<LA>Anglais</LA>
<EA>Commercial Optical Character Recognition (OCR) have at lot improved in the last few years. Their outstanding ability to process different kinds of documents is their main quality. However, their generality can also be an issue, as they cannot recognize perfectly documents far from the average present-day documents. We propose in this paper a system combining several OCRs and a specialized ICR (Intelligent Character Recognition) based on a convolutional neural network to complement them. Instead of just performing several OCRs in parallel and applying a fusing rule on the results, a specialized neural network with an adaptive topology is added to complement the OCRs, in function of the OCRs errors. This system has been tested on ancient documents containing old characters and old fonts not used in contemporary documents. The OCRs combination increases the recognition of about 3% whereas the ICR improves the recognition of rejected characters of more than 5%.</EA>
<CC>001D02B07B</CC>
<FD>Fouille donnée; Reconnaissance caractère; Reconnaissance optique caractère; Reconnaissance forme; Topologie circuit; Réseau neuronal; Méthode adaptative; Fonction erreur</FD>
<ED>Data mining; Character recognition; Optical character recognition; Pattern recognition; Network topology; Neural network; Adaptive method; Error function</ED>
<SD>Busca dato; Reconocimiento carácter; Reconocimento óptico de caracteres; Reconocimiento patrón; Red neuronal; Método adaptativo; Función error</SD>
<LO>INIST-16343.354000124412760710</LO>
<ID>05-0391622</ID>
</server>
</inist>
</record>
Pour manipuler ce document sous Unix (Dilib)
EXPLOR_STEP=$WICRI_ROOT/Ticri/CIDE/explor/OcrV1/Data/PascalFrancis/Corpus
HfdSelect -h $EXPLOR_STEP/biblio.hfd -nk 000449 | SxmlIndent | more
Ou
HfdSelect -h $EXPLOR_AREA/Data/PascalFrancis/Corpus/biblio.hfd -nk 000449 | SxmlIndent | more
Pour mettre un lien sur cette page dans le réseau Wicri
{{Explor lien
|wiki= Ticri/CIDE
|area= OcrV1
|flux= PascalFrancis
|étape= Corpus
|type= RBID
|clé= Pascal:05-0391622
|texte= Hybrid OCR combination for ancient documents
}}
| This area was generated with Dilib version V0.6.32. Data generation: Sat Nov 11 16:53:45 2017. Site generation: Mon Mar 11 23:15:16 2024 | 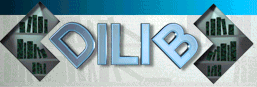 |